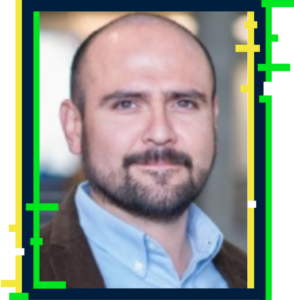
Alvaro Vazquez-Mayagoitia
Affiliation: Argonne National Laboratory
Country: IL, USA
Title: Machine learning potentials with ab initio accuracy in extreme scale environments
Abstract
With the availability of large amounts of computing power provided by distributed memory computers, hardware accelerators, and large datasets of quantum chemistry data, it has become possible to deploy accurate machine learning models that accelerate chemical discovery. These models achieve ab initio accuracy in a fraction of the computational cost. In this talk, I will discuss experiences using machine learning potentials (MLPs) as surrogate models to accelerate molecular dynamics simulations that study the thermodynamic properties of materials, specifically molten salts and 2D materials. I will cover the process of generating accurate data, distilling, training MLPs, and performing molecular dynamics at scale.
Bio
Álvaro Vazquez-Mayagoitia is a staff researcher in the Computational Science Division atArgonne National Laboratory and has worked on numerous projects to bring software and new algorithms to exascale computing at Argonne Leadership Computing Facility. Mayagoitia has successfully developed machine learning models to predict material properties.